代码:
# 导入必要的库
import numpy as np
import matplotlib.pyplot as plt
# 定义类1的数据点,每个数据点是二维的坐标
class1_points = np.array([[1.9, 1.2],
[1.5, 2.1],
[1.9, 0.5],
[1.5, 0.9],
[0.9, 1.2],
[1.1, 1.7],
[1.4, 1.1]])
# 定义类2的数据点,每个数据点是二维的坐标
class2_points = np.array([[3.2, 3.2],
[3.7, 2.9],
[3.2, 2.6],
[1.7, 3.3],
[3.4, 2.6],
[4.1, 2.3],
[3.0, 2.9]])
# 将两个类的数据点合并为一个大的数据集 x,标签合并为 y
x = np.concatenate((class1_points, class2_points), axis=0) # x 是一个 (14, 2) 形状的数组,包含所有的点
y = np.concatenate((np.zeros(len(class1_points)), np.ones(len(class2_points))), axis=0) # y 是一个包含标签的数组,0 表示 class1,1 表示 class2
# 计算先验概率,即每个类在数据集中的概率
prior_prob = [np.sum(y == 0) / len(y), np.sum(y == 1) / len(y)]
# 计算每个类的均值向量(每个类的特征的均值)
class_u = [np.mean(x[y==0], axis=0), np.mean(x[y==1], axis=0)]
# 计算每个类的协方差矩阵
class_cov = [np.cov(x[y==0], rowvar=False), np.cov(x[y==1], rowvar=False)]
# 定义概率密度函数 (PDF),用于计算高斯分布的概率
def pdf(x, mean, cov):
n = len(mean) # mean 是特征的维度,这里是2,因为每个数据点有两个特征
# 计算常数系数部分,注意是协方差矩阵的行列式
coff = 1 / (2 * np.pi) ** (n / 2) * np.sqrt(np.linalg.det(cov))
# 计算指数部分,(x - mean) 转置和协方差矩阵的逆相乘,再与 (x - mean) 相乘
exponent = np.exp(-(1 / 2) * np.dot(np.dot((x - mean).T, np.linalg.inv(cov)), (x - mean)))
# 返回高斯分布的概率密度
return coff * exponent
# 创建网格点,用于在平面上绘制决策边界
xx, yy = np.meshgrid(np.arange(0, 5, 0.05), np.arange(0, 4, 0.05))
# 将网格点转换为 (N, 2) 的矩阵,方便后续计算
grid_points = np.c_[xx.ravel(), yy.ravel()]
# 用于存储每个网格点的预测标签
grid_label = []
# 遍历网格中的每一个点,计算其后验概率,决定属于哪个类
for point in grid_points:
poster_prob = [] # 存储每个类的后验概率
for i in range(2): # 遍历两个类
# 计算每个类在该点的似然度(即高斯分布的概率)
likelihood = pdf(point, class_u[i], class_cov[i])
# 计算该类的后验概率,即 先验概率 * 似然度
poster_prob.append(prior_prob[i] * likelihood)
# 选择后验概率最大的类作为预测的标签
pre_class = np.argmax(poster_prob)
grid_label.append(pre_class)
# 绘制类1的样本点,蓝色标记
plt.scatter(class1_points[:, 0], class1_points[:, 1], c='blue', label='class 1')
# 绘制类2的样本点,红色标记
plt.scatter(class2_points[:, 0], class2_points[:, 1], c='red', label='class 2')
# 添加图例
plt.legend()
# 将 grid_label 转换为一个数组,并重塑为与网格形状一致的矩阵,便于绘制等高线图
grid_label = np.array(grid_label)
pre_grid_label = grid_label.reshape(xx.shape)
# 绘制决策边界,等高线图,绿色线表示决策边界(即类0与类1的分界线)
contour = plt.contour(xx, yy, pre_grid_label, levels=[0.5], colors='green')
# 显示绘图结果
plt.show()
结果:
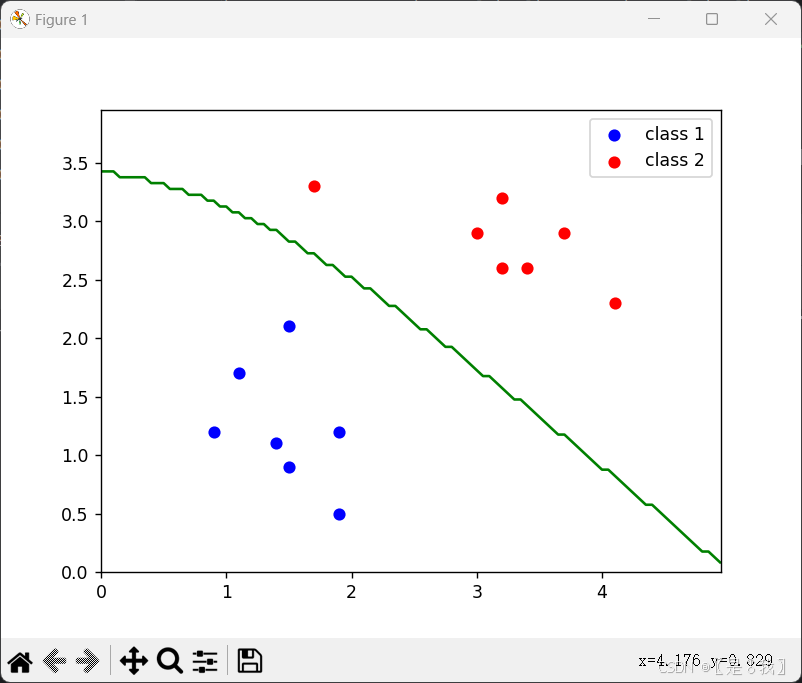